Business Problem
A large service company has inflated maintenance costs due to delayed detection of components that needed to be scrapped. This delay further affects new component procurement, thereby impacting maintenance turnaround time. The company would like to build a system for maintenance technicians to identify scrap as soon as they enter the maintenance facility.
Solution
The Scrap Detection System (SDS) used job logs entered by operators in order to identify relevant text/phrases that would indicate high probability of scrapping. Further, operational data recorded through sensors such as pressure and temperature and component physical parameters are added to the model for improving classification.
Features were extracted from the various data sources and a feature selection process was run to establish final set features to be used. A supervised learning algorithm (XG Boost) was then used in order to predict the probability of a component to be scrapped.
This system was deployed through a dashboard that indicated probability of scraping the component. The maintenance technician would then decide further course of action.

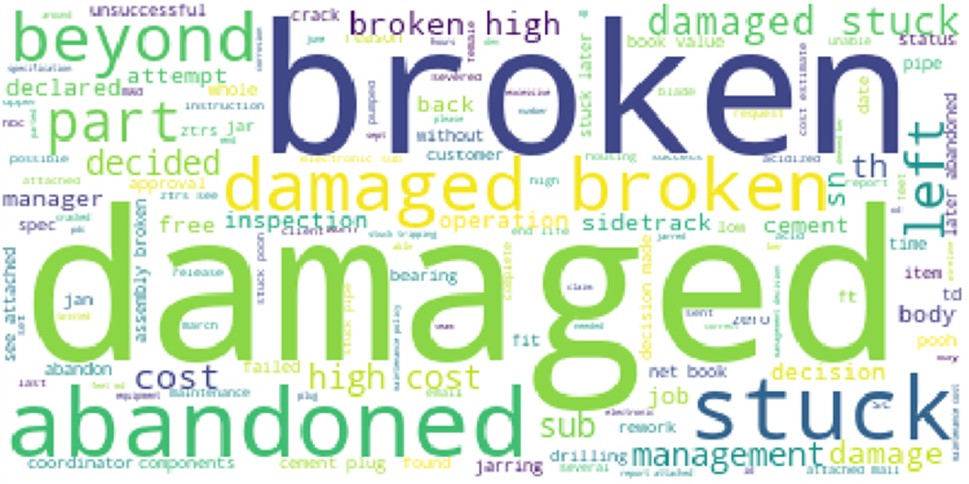
Impact
An accuracy of 72% was achieved in identifying scrap. This system helped the service function to improve lead times and also reduce rework cost on scrap components.
** The client has chosen AIDAS Analytics Office (subscription-based pay-as-you-go) services to run business analytics projects.